A recently published study explored various behavioral models to determine how an examiner’s proficiency and behavior influence their decisions when interpreting evidence.
Forensic science often involves comparing crime-scene evidence to a known-source sample to determine if the evidence and the reference sample came from the same source. While forensic analysis tools have become more objective, final source identification still relies on the examiner’s interpretation of the evidence. Each identification relies on judgments about features and the quality of the crime-scene evidence that may vary from one examiner to the next.
“Characterizing uncertainty in examiners’ decision-making has largely centered around proficiency tests or error rate studies without taking into account variations in behavior,” said Amanda Luby, an assistant professor of statistics at Swarthmore College and a Center for Statistics and Applications in Forensic Evidence (CSAFE) researcher.
Luby led the study with Anjali Mazumder, a Theme Lead at the Alan Turning Institute, and Brian Junker, a professor of statistics at Carnegie Mellon University.
The researchers proposed a new approach to account for differences among examiners and account for the varying difficulty among source identification tasks. Their approach was to use behavioral models from Item Response Theory (IRT) to understand the operating characteristics of the identification tasks that examiners perform. IRT is a family of mathematical models that attempt to explain the link between the properties of a test item and a person’s individual performance in response to it.
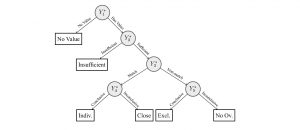
The study, published in Behaviormetrika, utilized the data from a 2011 FBI Black Box study of 169 fingerprint examiners that assessed the accuracy and reliability of the examiners’ decisions. The publicly available data included extra information about the decision-making process that Luby could use to apply IRT models.
“Applying IRT models to scored data provided a substantial improvement over error rates alone,” said Luby. “It allowed us to compare the proficiencies of the examiners even if they evaluated different pairs of prints.”
One of the models used by the researchers was Item Response Trees to visually represent the internal decision-making process an examiner goes through when analyzing a fingerprint. Luby said the Item Response Trees allowed for a more in-depth analysis of the factors involved in making a decision and a better understanding of the responses than simply judging them correct or incorrect.
Luby said the results from the study demonstrated the possibilities in accurately modeling the complex decision-making in fingerprint identification tasks.
“We anticipate future collaborations with experts in human decision making to improve the models and with fingerprint experts to determine the type and amount of data that would be needed to make precise and accurate assessments of examiner proficiency and task difficulty,” she said.
View and download the full journal article at https://lib.dr.iastate.edu/csafe_pubs/72/.
Additional Resources
Luby will give an in-depth review of this study at an upcoming CSAFE webinar. The webinar, IRT for Forensics: A Re-analysis of the FBI “Black Box Study,” will be held April 8 from 1-2 p.m. CST. The webinar is free and open to the public. To register, visit https://forensicstats.org/event/webinar-irt-for-forensics-a-re-analysis-of-the-fbi-black-box-study/.
Check out the Insights publication, Psychometric Analysis of Forensic Examiner Behavior.