Students participating in CSAFE’s 2023 Research Experience for Undergraduates (REU) program showcased their research projects at the Iowa State University Summer Undergraduate Research Symposium. The symposium was held Aug. 3 from 1-3 p.m. in the Memorial Union Great Hall.
The symposium provides an opportunity for all students engaged in research at Iowa State during the summer to come together and present their research in a poster format. For CSAFE’s students, the symposium was also the culmination of their eight-week immersive internship that provided invaluable experiences in forensic science research.
The four summer interns from CSAFE presented three posters at the symposium and were on hand to discuss their projects with the Iowa State community and the public. The posters are summarized below.
To learn more about the 2023 summer REU interns, check out this recent article: https://forensicstats.org/news-posts/a-new-cohort-of-reu-student-interns-bring-fresh-perspectives-to-toolmarks-footwear-and-digital-evidence-projects/.
Applications of Confocal Microscopes on Toolmark Analysis
Presenting Author: Eden Amin, a senior studying forensic science and criminal justice at the University of Central Oklahoma
Co-authors: Samantha Springer, a former CSAFE research assistant; Nate Simon, a recent graduate from Iowa State University; Sanika Gokakkar, a senior in mathematics at Iowa State University; Jeff Salyards, a CSAFE research scientist; and Heike Hofmann, a professor of statistics and professor-in-charge of data science at Iowa State University
View the poster at https://forensicstats.org/wp-content/uploads/2023/08/amin-2023reu.pdf.
Abstract: We lack published articles demonstrating the potential of wirecutter toolmark comparisons. This study involves creating test cuts with aluminum wire using Kaiweets wirecutters. Our preliminary findings show that test cuts have a small area of no striations, or “smush,” before leaving any striae. In the next steps, we will create a program to extract three-dimensional data, or a signature, from the test cuts, ultimately determining accuracy as a function of the area of the cut. For this study, we have created a method for achieving reliable scans of the aluminum wire on a confocal microscope. Our initial data collection, consisting of four wires cut per wire cutter at three separate locations (inner, middle, and outer), has shown some variance between the location of the test cut on the wire cutters and the scan itself. Statistical analysis of the cuts has proven there are distinguishing factors between wire cuts and being able to identify the replicate cuts. Each packaged wire has a corresponding file within an organized folder system to easily relocate and compare for future analysis.

Using Neural Networks to Identify Interest Points in Shoe Treads
Presenting Author: Matthew Nissen, a junior studying math at St. Olaf College
Co-author: Gautham Venkatasubramanian, a graduate student in statistics at Iowa State University
View the poster at https://forensicstats.org/wp-content/uploads/2023/08/nissen-2023reu.pdf.
Abstract: The goal of this project was to create a machine learning algorithm to detect corner points on pictures of shoe treads. Current corner detection software is only good at picking up on sharp, well-defined corners, which footprints rarely have. In the future, this type of technique could be used to aid further analysis by mapping the corner points onto shoe treads before more advanced techniques could work.
We first manually marked shoeprint photos with dots on the corners and sampled from these images to create our dataset of corner pixels and non-corner pixels. We then experimented with different neural network structures to optimize performance. We also ran many trials checking through hyperparameters, finding the optimal settings one by one. Examples of these hyperparameters are learning rates, number of training cycles, image size, and layer numbers.
The project was largely successful, creating a model with over 90% accuracy on data it had never seen before. We found that simple models worked best overall, allowing for strong outcomes while preventing overfitting and speeding up computation times.
This model will need to be continuously improved, however, because the pictures contain thousands of pixels, and marking 10% of them incorrectly means hundreds of wrong marks. What we have now will work as a powerful starting point for this future research.
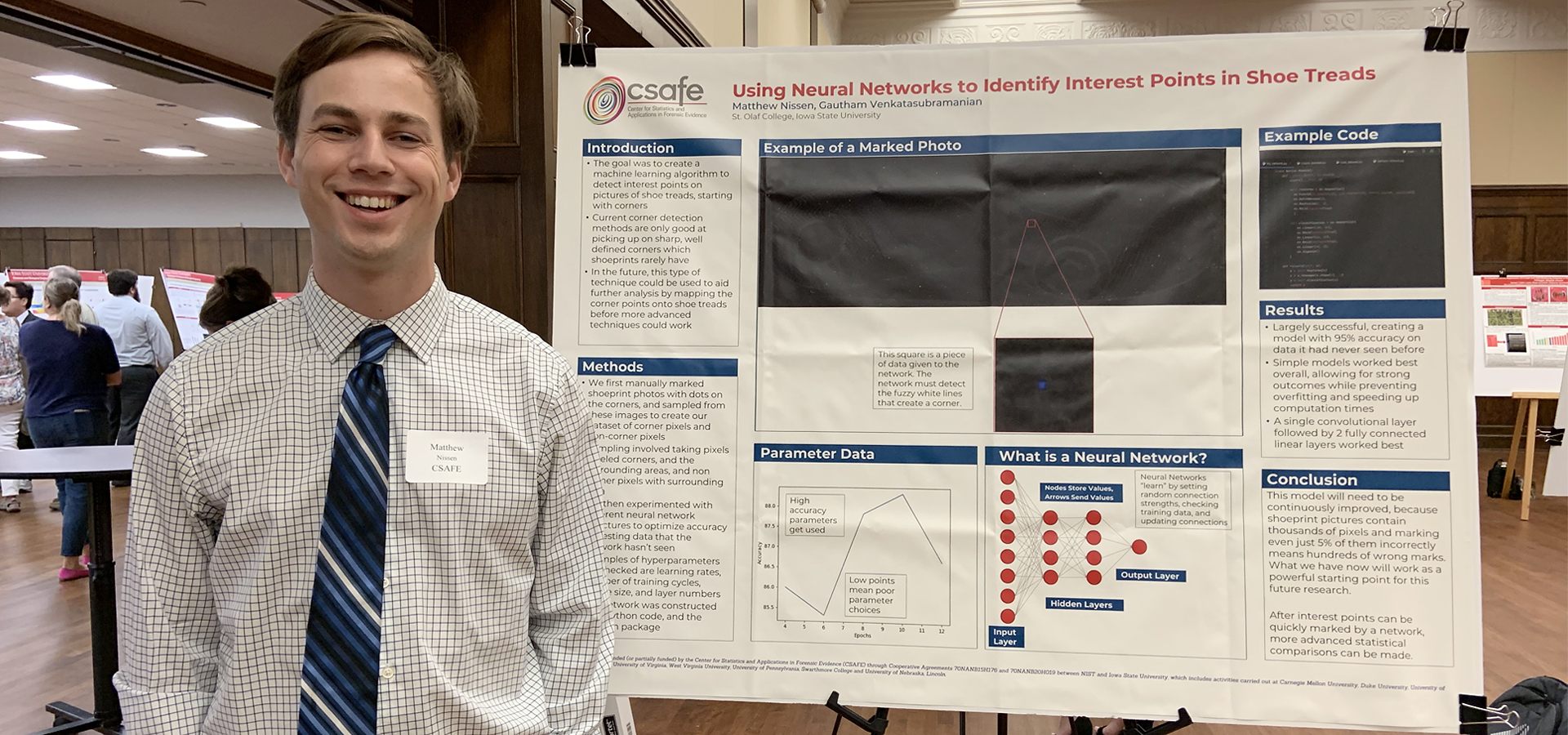
Expanding ShoeCase: A Mock Crime Scene Footwear Impression Database
Presenting Authors: Saniya Lyles, a forensic science major at Fayetteville State University, and Tiffany Ongtowasruk, a senior in general biology and criminology and justice studies at Fort Lewis College
Co-authors: Abigail Tibben, a senior in genetics at Iowa State University, and Gautham Venkatasubramanian, a graduate student in statistics at Iowa State University
View the poster at https://forensicstats.org/wp-content/uploads/2023/08/lyles-2023reu.pdf.
Abstract: One of the continuous problems in forensic science is a lack of data. Creating datasets representative of casework is a challenge because it can be difficult to obtain large quantities of images collected under the same protocol and with the same shoes. Data collected in this way, however, can be of significant use for researchers and training within forensic science disciplines.
The current ShoeCase dataset is active on ISU Datashare, with mixed impression types, flooring, lift techniques, and digital file types. The complete dataset will include over 900 shoeprint images contained in more than 3,000 digital files. While this allows further research in forensics, there were only two shoe types for these impressions. The goal is to make this dataset as robust and authentic as possible, which will allow for higher levels of testing and certainty with current and future research.
The REU team at the Center for Statistics and Applications in Forensic Evidence (CSAFE) worked to expand the data that we currently have with new types of shoes and alternate collection protocols. Our poster presentation will explain why this dataset is important, the process of collecting and processing images, as well as the importance of documented variability within datasets.
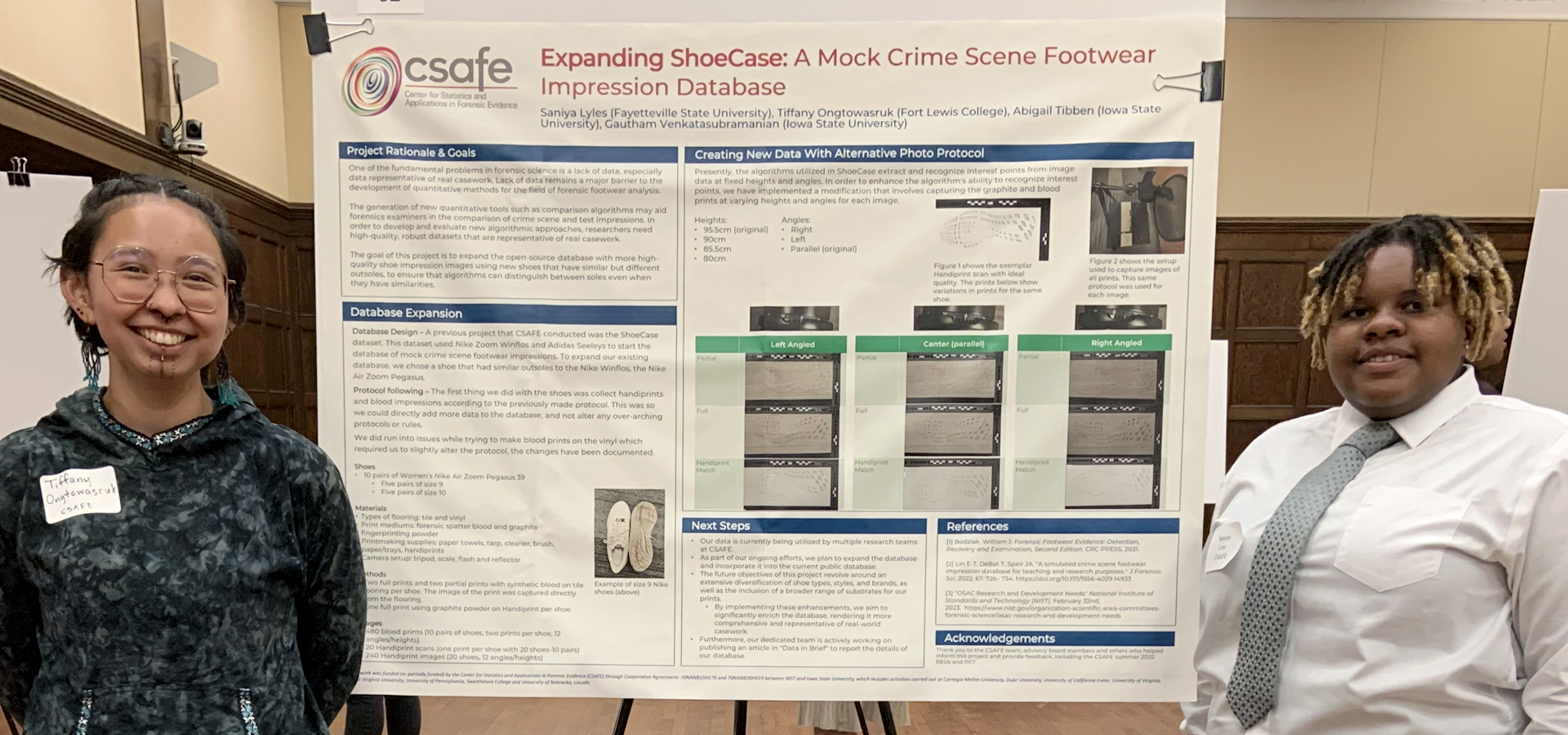